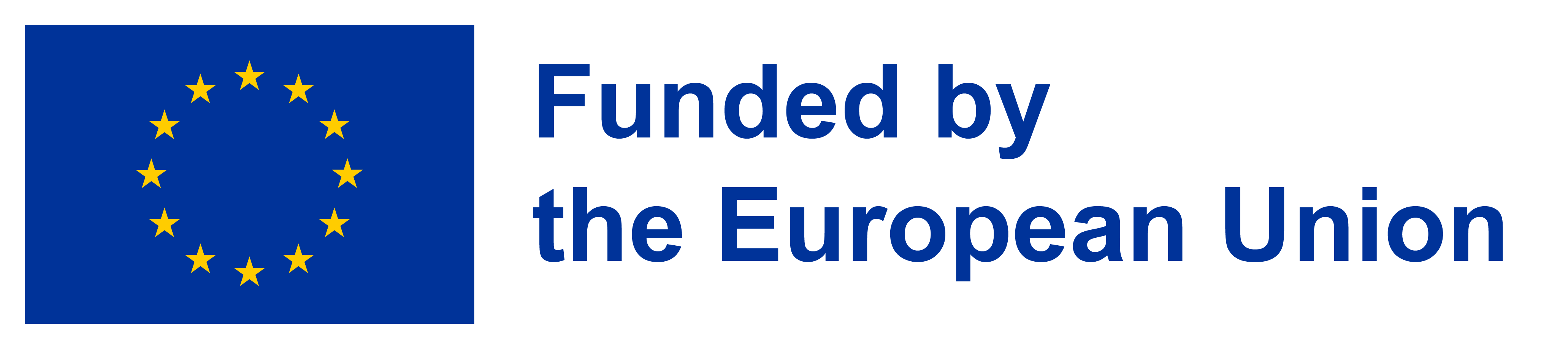
-
Natural sciences
- Astronomical and space instrumentation
Though Big Data has become common in many domains nowadays, the challenges to develop efficient and automated mining of the ever increasing data sets by new generations of data scientists are eminent. These challenges span wide swathes of society, business and research. Astronomers with their high-tech observatories are historically at the forefront of this field, but obviously, the impact in e.g. commercial applications, security, environmental monitoring and experimental research is immense. We aim to contribute to this general discussion by training a number of young scientists in the fields of computer science and astronomy, focussing on techniques of automated learning from large quantities of data to answer fundamental questions on the evolution of properties of galaxies. While these techniques will lead to major advances in our understanding of the formation and evolution of galaxies, we will also promote, in collaboration with industry, much more general applications in society, e.g. in medical imaging or remote sensing. We have put together a team of astronomers and computer scientists, from academic and private sector partners, to develop techniques to detect and classify ultra-faint galaxies and galaxy remnants in a deep survey of the Fornax cluster, and use the results to study how galaxies evolve in the dense environment of galaxy clusters. With a team of young researchers we will develop novel computer science algorithms addressing fundamental topics in galaxy formation, such as the huge dark matter fractions inferred by theory, and the lack of detected angular momentum in galaxies. The collaboration is unique - it will develop a platform for deep symbiosis of two radically different strands of approaches: purely data-driven machine learning and specialist approaches based on techniques developed in astronomy. Young scientists trained with such skills are highly demanded both in research and business.