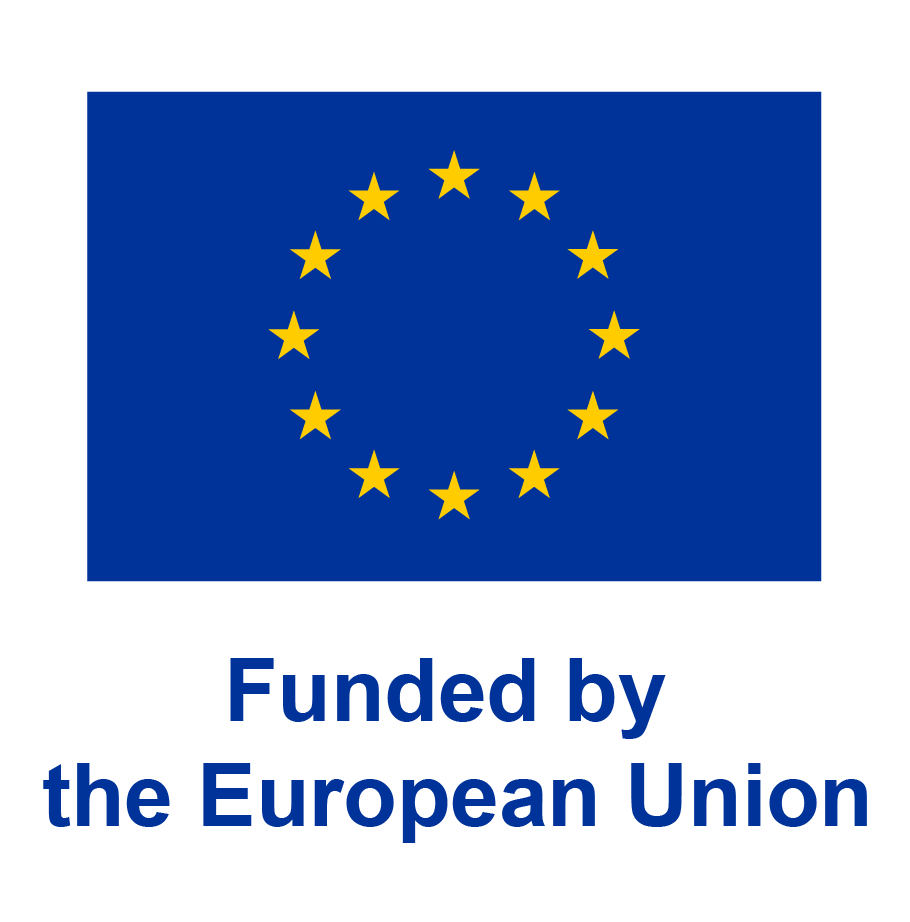
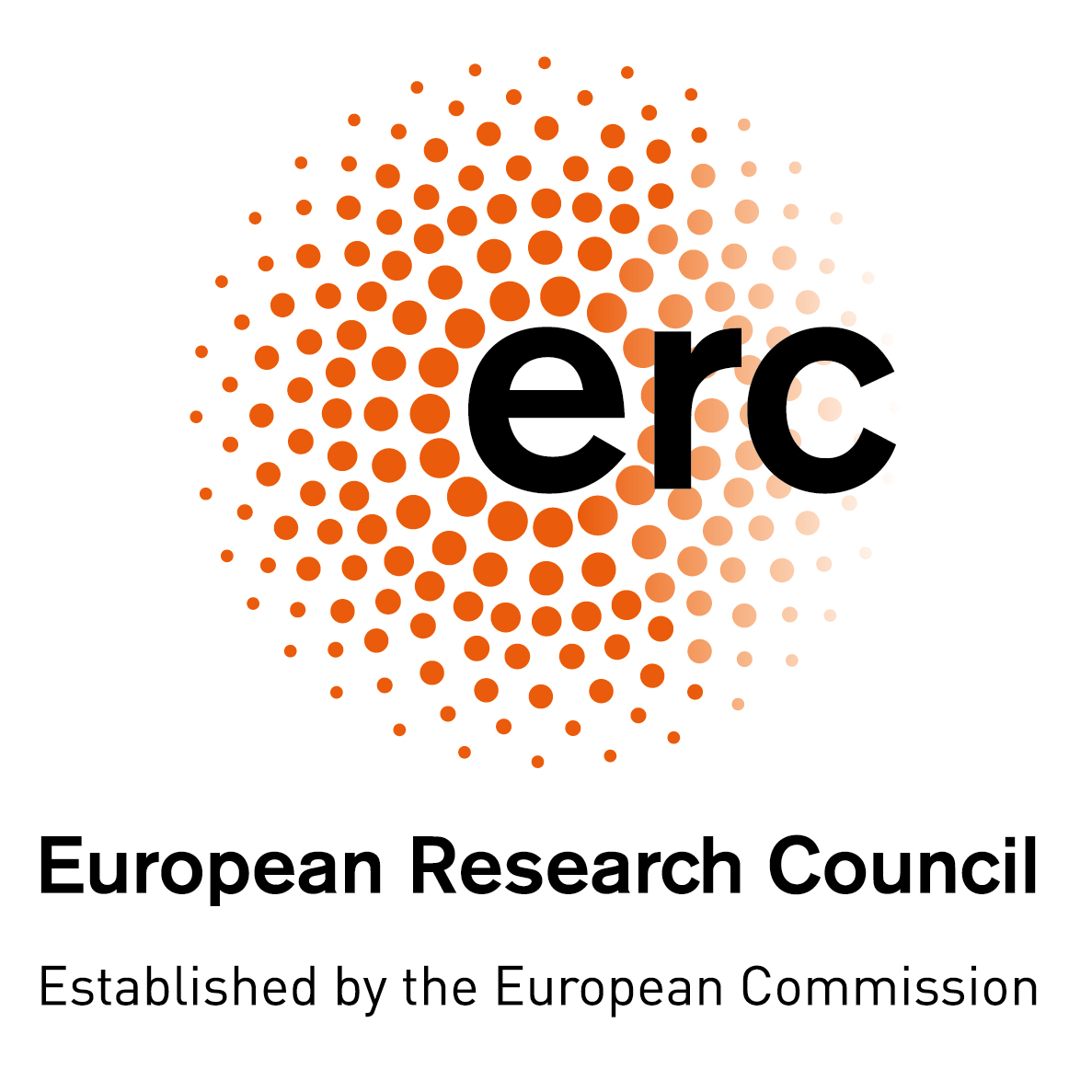
-
Natural sciences
- Statistics not elsewhere classified
- Machine learning and decision making
-
Social sciences
- Causes and prevention of crime
- Criminography and methods of criminological investigation
- Police administration, procedures and practice
- Safety, prevention and police
- Human rights law
Big data policing is an innovative strategy that uses historical data to forecast when and where there is a high risk of new crime events in order to use police resources more efficiently and proactively, and ultimately reduce crime rates. Big data policing models can consist of variables based on crime data available in police databases (e.g. previous crime events), socio-economic data (e.g. poverty index, residential mobility), opportunity characteristics (e.g. the presence of shops, distance to nearest highway), data from new technologies (e.g. intelligent cameras) and other known predictors of crime (e.g. police patrol intensity).
The overarching objective of this ERC project is to unite and integrate the statistical-methodological, criminological, legal and ethical dimensions of big data policing in an evidence-based model that will be tested by different randomized controlled trials and built on the principles of an international (i.e. European) and interdisciplinary approach.