Acronym
AMICAS
Code
41S07822
Duration
01 October 2022 → 30 September 2027
Funding
European funding: framework programme
Principal investigator
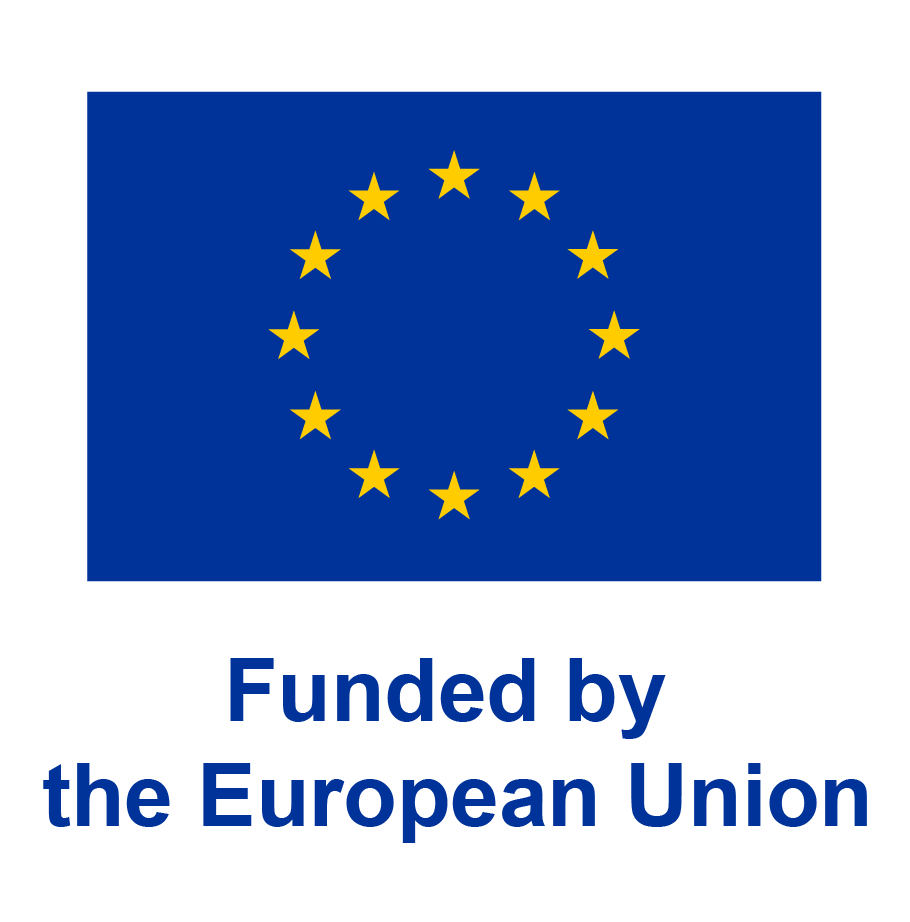
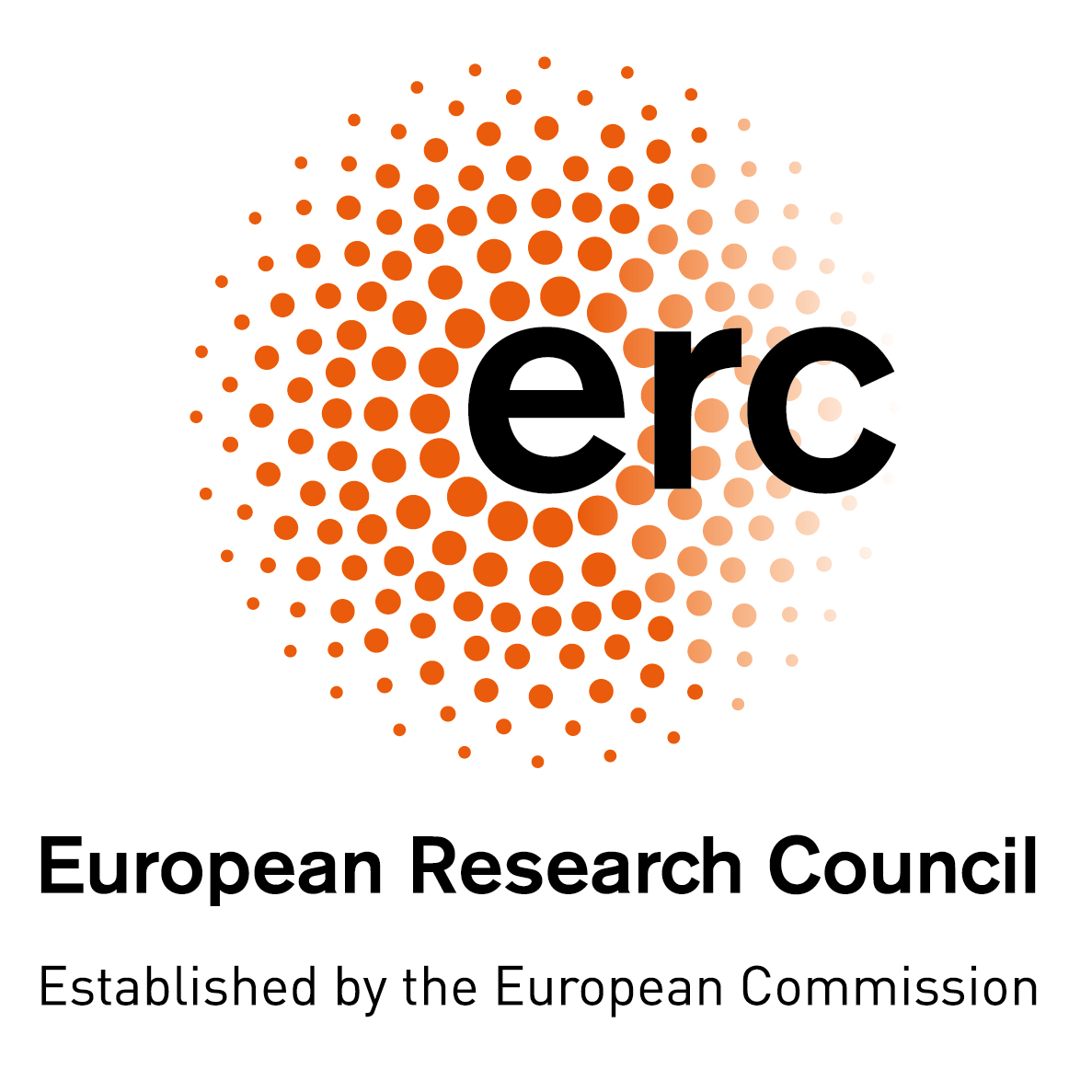
Research disciplines
-
Engineering and technology
- Automation and control systems
- Signal processing
- Biomedical modelling
Keywords
systems and control
Bioelectronics
biomedical engineering and instrumentation
analytic modelling
Other information
Project description
A major challenge in anesthesia is to adapt the drug infusion rates from observed patient response to surgical stimuli. The patient models are based on nominal population characteristic response and lack specific surgical effects. In major surgery (e.g. cardiac, transplant, obese patients) modelling uncertainty stems from significant blood losses, anomalous drug diffusion, drug effect synergy/antagonism, anesthetic-hemodynamic interactions, etc. This complex optimisation problem requires superhuman abilities of the anesthesiologist.
Computer controlled anesthesia holds the answer to be the game changer for best surgery outcomes. Although few, clinical studies report that computer based anesthesia for one or two drugs outperforms manual management. In reality, clinical practice mitigates a multi-drug optimization problem while accommodating large patient model uncertainty. The anesthesiologist makes decisions based on future surgeon actions and expected patient response. This is a predictive control strategy, a mature methodology in systems and control engineering with potential to faster recovery times and lower risk of complications. The goal of AMICAS is to advance the scope and clinical use of computer based constrained optimization of multi-drug infusion rates for anesthesia with strong effects on hemodynamics. We plan to identify multivariable models and minimize the large uncertainties in patient response. With adaptation mechanisms from nominal to individual patient models, we design multivariable optimal predictive control methodologies to manage strongly coupled dynamics. To maximize performance of the closed loop, we model the surgical stimulus as a known disturbance signal and additional bolus infusions from anesthesiologist as known inputs. Integrating human expertise with computer optimization provides a successful solution for breakthrough into clinical practice.
Role of Ghent University
Ghent University is the host institution for this project, endorsing groundbreaking research and providing full administrative and infrastructure support, with special care for psychosocial well-being environment of its employees.
Disclaimer
Funded by the European Union. Views and opinions expressed are however those of the author(s) only and do not necessarily reflect those of the European Union or the European Research Council Executive Agency (ERCEA). Neither the European Union nor the authority can be held responsible for them.