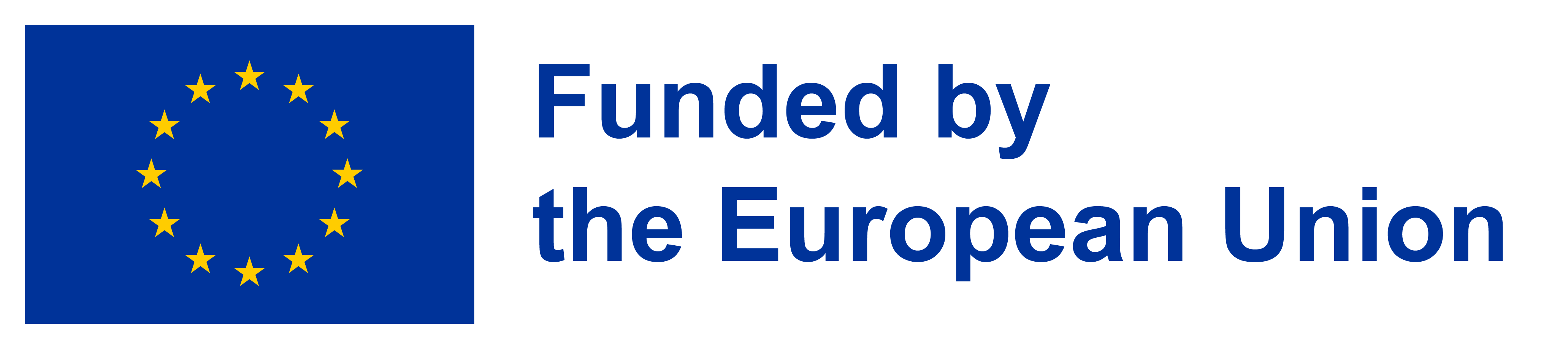
-
Social sciences
- Motivation and emotion
Our visual system constantly encounters a plethora of stimuli, drastically surpassing our processing capabilities for in-depth analysis. It is hence essential to select the element(s) that is most relevant for our objectives and ignore irrelevant ones. One helpful feature for this is that the world is characterized by numerous regularities making it partially predictable. Our brain can extract these regularities from past experience and use them to efficiently guide target selection. Recently, significant scientific interest has been directed to two forms of learning that focus on two types
of regularities: in reward-mediated learning (RL), people learn which stimuli are associated with reward, prioritizing the elements related with high (vs. low) reinforcement. Statistical learning (SL), in contrast, allows people to extract statistical regularities from the environment, such as how often a stimulus occurs in a specific location, optimizing future actions toward the location where the relevant element appears frequently. In everyday life RL and SL coexist and they jointly guide our selection of relevant stimuli. However, they have been mainly addressed separately using divergent tasks, hindering a direct comparison of the results and assessing their combined influence. This project seeks to bridge this gap by systematically comparing RL and SL in a systematic and well-balanced experimental setup to understand how people implicitly learn from their past experience. Using a series of consistent experimental tasks, behavioral, ocular and electroencephalography measures will be recorded from healthy human volunteers to assess the individual and joint effect of RL and SL on the process dynamics from target selection until response execution. Furthermore, employing Markov decision process models, we will compare the observed performance with model performance. Jointly, our results will provide important insights into real-world learning mechanisms.